Empowering Machine Learning with Labeled Insights
Data annotation technology stands as a pivotal component in the realm of machine learning (ML) and artificial intelligence (AI), providing the means to enrich raw data with contextual information. This process involves labeling or tagging datasets to facilitate the training and development of ML models. This article explores the intricacies of data annotation technology, its significance, and its role in empowering advanced AI applications.
Fundamentals of Data Annotation:
- Data annotation involves the systematic labeling or tagging of data to enhance its interpretability by machine learning algorithms.
- Annotation provides crucial context and identification for diverse data types, including images, text, audio, and more.
- Types of Annotations:
- Various types of annotations cater to different data modalities. Image data may be annotated with bounding boxes, segmentation masks, or keypoint annotations.
- Text data annotation encompasses categorization, sentiment analysis labels, and named entity recognition, among other tasks.
- Human-in-the-Loop Annotation:
- Human annotators often play a central role in data annotation, especially for tasks requiring nuanced understanding, context, or subjective judgment.
- Human-in-the-loop annotation ensures the incorporation of human expertise in complex labeling scenarios.
- Quality Control and Annotation Guidelines:
- Establishing robust annotation guidelines is essential for maintaining data quality and consistency.
- Quality control processes help identify and rectify errors, ensuring that annotated datasets accurately represent the ground truth.
- Automated Annotation Tools:
- Advancements in technology have led to the development of automated annotation tools. Computer vision algorithms and natural language processing models contribute to automating annotation tasks.
- Automation accelerates the annotation process, reduces human labor, and enhances efficiency.
- Applications Across Industries:
- Data annotation finds application in diverse sectors, including image recognition, object detection, natural language processing, autonomous vehicles, and medical image analysis.
- Annotated datasets serve as the foundation for training ML models to perform tasks ranging from identifying objects in images to extracting insights from textual content.
- Challenges and Considerations:
- Data annotation can be resource-intensive, particularly for large and intricate datasets.
- Addressing challenges such as ensuring accuracy, managing subjective labeling tasks, and dealing with evolving data types are critical considerations in the annotation process.
In the dynamic landscape of AI and machine learning, data annotation technology emerges as a linchpin, bridging the gap between raw data and the intelligence of ML algorithms. Its role in contextualizing and labeling datasets paves the way for the development of accurate and robust models across various domains. As advancements continue, data annotation technology is set to be a driving force in unlocking the full potential of AI applications, propelling us into a future where machines can comprehend, learn, and make informed decisions with unprecedented depth and accuracy.
The Reality of Data Annotation Technology: Bridging the Gap in Machine Learning
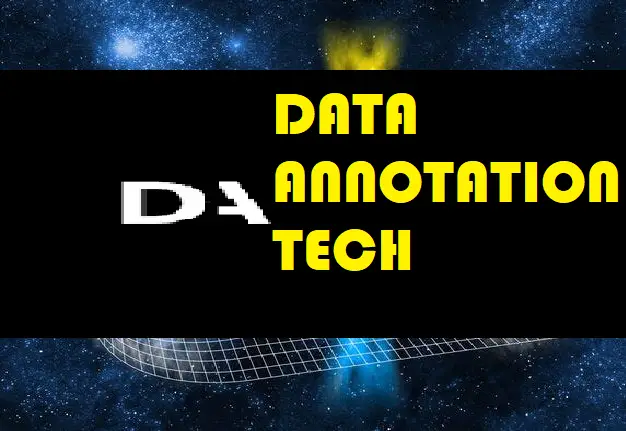
Data annotation technology, a crucial facet of the ever-evolving landscape of artificial intelligence (AI) and machine learning (ML), is indeed very real. This technology is legit and very real. Its significance lies in its ability to transform raw data into comprehensible and trainable datasets for machine learning models. This article delves into the tangible reality of data annotation technology, exploring its functionalities, real-world applications, and the impact it has on the advancement of AI.
- Defining Data Annotation Technology:
- Data annotation technology is not a mere concept but a practical and essential process in ML and AI development.
- It involves the meticulous labeling, tagging, or annotating of datasets to impart contextual information for training machine learning models.
- Real-world Applications:
- The reality of data annotation technology is vividly demonstrated through its widespread applications across industries.
- In image recognition, annotation tools label objects within images, guiding ML models to recognize and classify them accurately.
- Text annotation aids in tasks like sentiment analysis, where human annotators label text data to train models in understanding the emotional tone of a piece of text.
- Human-in-the-Loop Annotation:
- Human involvement in data annotation is a testament to its reality. Human-in-the-loop annotation is crucial for tasks that require nuanced understanding, subjective judgment, and contextual interpretation.
- Humans contribute their expertise to ensure the quality and relevance of labeled data, further emphasizing the practicality of data annotation technology.
- Quality Control Mechanisms:
- The reality of data annotation technology is also evident in the meticulous quality control mechanisms established to maintain data accuracy.
- Annotation guidelines, continuous monitoring, and feedback loops are implemented to rectify errors and ensure that the annotated datasets align with the ground truth.
- Automated Annotation Tools:
- Advancements in technology have given rise to automated annotation tools, showcasing the real-world evolution of data annotation.
- Computer vision algorithms and natural language processing models contribute to automating tasks, reducing manual labor, and increasing the efficiency of the annotation process.
- Tangible Impact on Machine Learning Models:
- The real impact of data annotation technology is seen in the improved performance of machine learning models.
- Annotated datasets serve as the foundation for training models, enabling them to recognize patterns, make predictions, and perform tasks with a level of accuracy that wouldn’t be achievable without annotated reference points.
- Addressing Challenges:
- The reality of data annotation technology is not without challenges. Issues such as resource intensity, subjective labeling tasks, and managing evolving data types are acknowledged and actively addressed within the industry.
Data annotation technology is not a speculative concept but a tangible and indispensable component of modern machine learning and artificial intelligence. Its impact is felt across industries, from image and text annotation to real-world applications like autonomous vehicles and medical image analysis. As the technology continues to evolve, data annotation remains a dynamic force, propelling machine learning models toward unprecedented levels of accuracy, understanding, and applicability in diverse domains. Its reality underscores the transformative potential of annotated data in shaping the future of AI.
Early adopters of the tech
Current Adopters of Data Annotation Technology: A Cross-Industry Overview
Data annotation technology has transcended its theoretical roots to become a cornerstone of various industries, where its practical applications are transforming the landscape of artificial intelligence and machine learning. This article explores the diverse range of industries and entities that are actively using data annotation technology today.
- Tech Giants and Research Institutions:
- Leading technology companies, including giants like Google, Microsoft, and Facebook, heavily rely on data annotation technology for training robust machine learning models.
- Research institutions and laboratories leverage annotation tools to label datasets, contributing to advancements in computer vision, natural language processing, and other AI-related fields.
- Automotive Industry:
- Companies within the automotive sector, such as Tesla, Ford, and General Motors, employ data annotation technology for developing autonomous vehicles.
- Image and video annotation play a crucial role in training AI systems to recognize and respond to diverse road scenarios and obstacles.
- E-commerce and Retail:
- E-commerce platforms, like Amazon and Alibaba, utilize data annotation for image recognition in product cataloging and recommendation systems.
- Retailers leverage annotation technology for tasks such as visual search, ensuring accurate and efficient product matching based on user queries.
- Healthcare and Medical Imaging:
- In the healthcare industry, data annotation is integral for medical image analysis. Companies like Siemens Healthineers and GE Healthcare use annotated datasets to train models for detecting abnormalities in medical images.
- Annotation aids in the development of AI applications for early disease diagnosis and treatment planning.
- Agriculture and Precision Farming:
- Agriculture companies, such as John Deere, employ data annotation for precision farming applications.
- Image annotation helps in identifying crop health, monitoring growth patterns, and implementing precision agriculture techniques for optimized yield.
- Financial Services:
- Financial institutions utilize data annotation for natural language processing tasks, sentiment analysis, and fraud detection.
- Annotated text data contributes to training models that can analyze market trends, customer sentiment, and detect unusual patterns indicative of fraudulent activities.
- Telecommunications and Communication Networks:
- Telecom companies like AT&T and Verizon employ data annotation technology for network optimization and fault detection.
- Annotation of network data helps in training models to identify and predict potential issues, ensuring robust and reliable communication networks.
- Social Media Platforms:
- Social media platforms, including Instagram, Facebook, and Twitter, utilize data annotation for image and content moderation.
- Annotation helps in identifying and filtering content that violates community guidelines, ensuring a safer online environment.
Tools of the trade
Exploring Data Annotation Tools: Enabling Precision in Machine Learning
Data annotation tools have become indispensable in the realm of machine learning, facilitating the process of labeling and enriching datasets for training AI models. This article provides an in-depth exploration of data annotation tools, discussing their functionalities, types, and the pivotal role they play in enhancing the accuracy of machine learning algorithms.
- Overview of Data Annotation Tools:
- Data annotation tools are software solutions designed to assist in the labeling, tagging, or annotating of datasets.
- These tools streamline the process of preparing data for machine learning tasks, making it understandable and trainable for AI models.
- Image Annotation Tools:
- Image annotation tools are crucial for tasks involving visual data. Bounding box annotation tools, such as Labelbox and VGG Image Annotator (VIA), allow annotators to draw bounding boxes around objects in images.
- Semantic segmentation tools, like RectLabel and Supervisely, enable the pixel-wise annotation of images, providing detailed information about object boundaries.
- Text Annotation Tools:
- Text annotation tools are employed for tasks involving natural language processing (NLP). Prodigy, Brat, and Doccano are examples of tools that facilitate text categorization, sentiment analysis, and named entity recognition.
- These tools allow annotators to highlight and categorize specific elements within text data, enhancing the understanding of language patterns by machine learning models.
- Audio Annotation Tools:
- Audio annotation tools cater to tasks involving sound data. Tools like Audacity and Praat enable annotators to mark specific segments in audio files, such as identifying spoken words or distinguishing between different sounds.
- These tools contribute to training models for speech recognition, acoustic analysis, and other audio-related applications.
- Video Annotation Tools:
- Video annotation tools, such as Annotator and VGG Video Annotator (VIVA), allow annotators to mark objects or events within video sequences.
- These tools are essential for training machine learning models in video analysis, object tracking, and action recognition.
- 3D Point Cloud Annotation Tools:
- For tasks involving three-dimensional data, 3D point cloud annotation tools like Pointfuse and CloudCompare enable annotators to label objects in a three-dimensional space.
- These tools are crucial for applications like autonomous vehicle development and augmented reality.
- Collaborative Annotation Platforms:
- Collaborative annotation platforms, such as Labelbox and Supervisely, facilitate teamwork in the annotation process.
- These tools provide a centralized environment where multiple annotators can work on the same dataset simultaneously, ensuring consistency and quality in the annotations.
- Automated Annotation Tools:
- Advancements in technology have led to the development of automated annotation tools that use machine learning algorithms to assist in the annotation process.
- These tools, integrated into platforms like Snorkel and LightTag, help speed up the annotation workflow by automating repetitive tasks and reducing manual labor.
Data annotation tools have evolved into sophisticated solutions catering to various data types and machine learning tasks. From image and text annotation to audio and video annotation, these tools play a pivotal role in preparing datasets for machine learning models. As technology continues to advance, data annotation tools are expected to become even more sophisticated, further streamlining the annotation process and contributing to the development of highly accurate and efficient AI models.
Data Annotation Jobs: A Perspective on Remote Working or Working from Home
The field of data annotation has witnessed a significant transformation, not only in terms of technology but also in the way jobs within this sector are structured. This article provides a comprehensive perspective on data annotation jobs, focusing on the increasing prevalence and advantages of remote working or working from home in this domain.
- Evolution of Data Annotation Jobs:
- Traditionally, data annotation jobs were often performed on-site, requiring annotators to be physically present in a designated workspace.
- With advancements in technology and the rise of cloud-based annotation platforms, remote working has become increasingly feasible and prevalent in the field of data annotation.
- Remote Working in Data Annotation:
- Remote working or working from home has become a viable option for data annotators, offering flexibility and the ability to work from any location.
- Annotators can access cloud-based annotation tools and collaborate with teams in real-time, breaking down geographical barriers and allowing for a more diverse and globally distributed workforce.
- Advantages of Remote Data Annotation Jobs: a. Flexibility:
- Remote working provides annotators with the flexibility to choose their working hours, accommodating different time zones and personal schedules. b. Cost Savings:
- Eliminating the need for a physical office space reduces overhead costs for companies, while annotators save on commuting expenses. c. Access to a Global Talent Pool:
- Remote working allows companies to tap into a diverse talent pool worldwide, enabling them to hire annotators with specific expertise or language skills.
- Challenges and Solutions: a. Communication and Collaboration:
- Maintaining effective communication and collaboration can be challenging in remote settings. However, the use of collaboration tools, video conferencing, and project management platforms helps bridge this gap. b. Security and Confidentiality:
- Ensuring data security and confidentiality is paramount. Companies employ secure cloud-based annotation platforms and implement strict privacy measures to address these concerns.
- Training and Onboarding:
- Remote working requires effective strategies for training and onboarding new annotators. Virtual training sessions, detailed documentation, and mentorship programs are essential to ensure a smooth onboarding process.
- The Role of Technology:
- Cloud-based annotation tools and platforms play a crucial role in facilitating remote data annotation jobs. These tools enable annotators to access datasets, collaborate with team members, and perform annotation tasks seamlessly from any location.
- Job Satisfaction and Work-Life Balance:
- Remote working contributes to job satisfaction by providing annotators with a better work-life balance. This flexibility can lead to increased productivity, job retention, and overall job satisfaction.
- Future Outlook:
- The future of data annotation jobs is likely to see a continued rise in remote working arrangements, with companies recognizing the benefits of a flexible and globally distributed workforce.
- Advancements in virtual collaboration tools and security measures will further support the growth of remote data annotation jobs.
The landscape of data annotation jobs is undergoing a significant shift towards remote working or working from home. This transformation not only offers flexibility and cost savings but also enables companies to access a global talent pool. With the right technology and strategies in place, remote data annotation jobs are poised to become an integral and sustainable aspect of the evolving workforce in this dynamic field.
I hope with the current article it must be really clear about what is data annotation technology? What is meant by data annotation technology?
Data annotation technology has permeated a wide spectrum of industries, from technology giants and healthcare to agriculture and finance. Its adoption underscores the essential role it plays in enhancing the capabilities of machine learning models across diverse applications. As industries continue to leverage data annotation, we can anticipate further advancements in AI, leading to innovative solutions and improved efficiencies across various sectors.
Very good written post. It will be helpful to anybody who usess it, including myself. Keep up the good work – i will definitely read more posts.
Wow, awesome blog layout! How long have you been blogging for? you make blogging look easy. The overall look of your website is fantastic, let alone the content!
I’m still learning from you, as I’m improving myself. I certainly liked reading all that is posted on your site.Keep the information coming. I loved it!
you might have a great blog here! would you prefer to make some invite posts on my weblog?
I think this is one of the most important information for me. And i am glad reading your article. But want to remark on some general things, The website style is great, the articles is really excellent : D. Good job, cheers
I ‘d mention that most of us visitors are endowed to exist in a fabulous place with very many wonderful individuals with very helpful things.
You completed several good points there. I did a search on the subject and found most persons will have the same opinion with your blog.
It has been simply incredibly generous with you to provide openly what exactly many individuals would’ve marketed for an ebook to end up making some cash for their end, primarily given that you could have tried it in the event you wanted.
Many thanks to you for sharing these types of wonderful content. In addition, the ideal travel and medical insurance strategy can often eradicate those considerations that come with traveling abroad. Any medical emergency can before long become costly and that’s guaranteed to quickly set a financial load on the family’s finances. Putting in place the great travel insurance offer prior to leaving is well worth the time and effort. Thank you
A person essentially lend a hand to make critically posts I would state. This is the very first time I frequented your web page and thus far? I amazed with the analysis you made to make this particular submit amazing. Great activity!
hello there and thank you for your information ?I抳e certainly picked up something new from right here. I did however expertise a few technical issues using this web site, since I experienced to reload the web site many times previous to I could get it to load properly. I had been wondering if your web hosting is OK? Not that I’m complaining, but sluggish loading instances times will sometimes affect your placement in google and could damage your high-quality score if advertising and marketing with Adwords. Anyway I抦 adding this RSS to my email and could look out for much more of your respective intriguing content. Make sure you update this again very soon..
Here is the host that I am using this is my referral link as well – HOSTINGER – https://cart.hostinger.com/pay/8fc99ad8-3eb2-4203-8ad3-0ef9832ad30b?_ga=GA1.3.942352702.1711283207
I have been surfing online greater than 3 hours nowadays, but I by no means discovered any interesting article like yours. It is pretty worth enough for me. In my opinion, if all site owners and bloggers made good content as you probably did, the net can be much more useful than ever before.